Margin of Inefficiency in Prediction Markets
Prediction market price disparities can be explained by well-understood structural factors
Despite well-established methodology and long history in politics, polling errors have become common, if not expected, in recent election cycles. As the figure below shows, polls for the 2020 presidential election underestimated Republican support in 48/50 states.
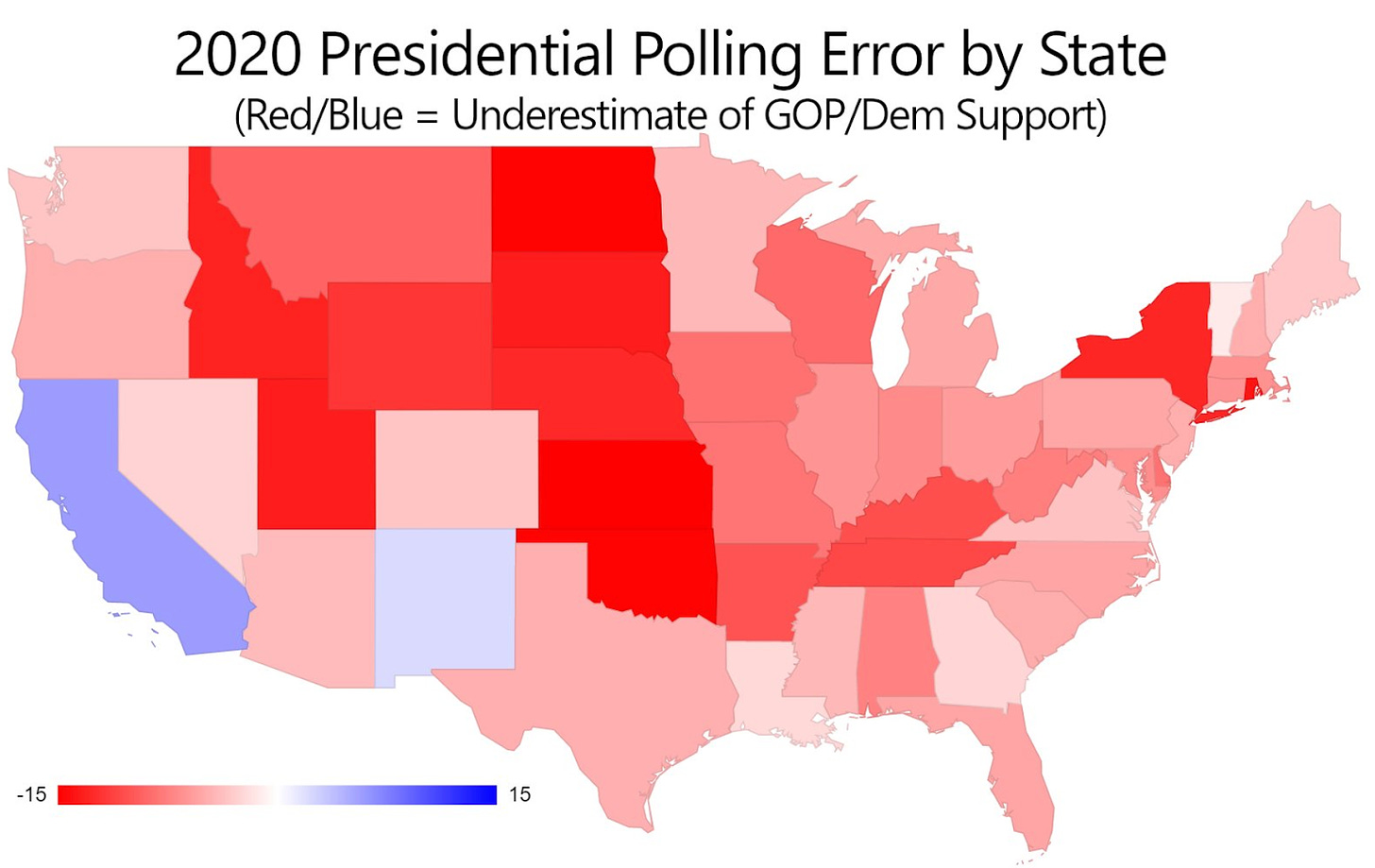
In parallel to the public’s declining trust in traditional polling, political betting markets have risen in popularity to fill the void. Research on the 2018 and 2020 election cycles suggests that political prediction markets are more accurate than polls. That research was based on a comparison between the poll aggregation at FiveThirtyEight and the prediction market at PredictIt. In fact, that research likely understates the relative accuracy of prediction markets because it compares the most respected source for poll aggregation (FiveThirtyEight) with a small-scale, low volume betting market (PredictIt).
Just as not all polling results are of equal quality, not all betting markets are of equal accuracy. In polling, the primary measure of quality is statistical error, as measured by the margin of error. In betting markets, the primary source for inaccuracy is inefficiency, as measured by what I call the margin of inefficiency.
In opinion polling the margin of error reflects the relative accuracy of a poll result based on the sampling methodology and established statistical theory. Under basic sampling theory, the margin of error of a poll with N participants is reported as 1 / sqrt(N). So, for a sample with 1000 participants, the margin of error is 3.2%, for 2000 participants is 2.2%, and for 5000 participants is 1.4%. The fact that many polls report a margin of error of about 3% reflects that the standard sample size for political polling is around 1,000 participants.
In political polling, when one candidate receives 1% more support than another in a poll with a 3.0% margin of error, the result is said to be “within the margin of error”, and is sometimes referred to as a “statistical tie”. Similarly, two polls, both with a 3.0% margin of error, with one reporting candidate A as +1.0% and the other reporting candidate B as +1.0%, are said to be within the margin of error of each other.
The concept of margin of error in statistical polling underscores the uncertainty inherent in opinion polling, and communicates that two polls whose results are different can nevertheless be consistent with the same underlying reality. In the above example, both polls are consistent with the reality that candidate A is +2.0%, even though one reports +1.0% and the other -1.0% for that candidate. Both polls are also consistent with the reality that candidate A is -2.0%. In fact, technically these polls are consistent with any result, even a large outlying result such as candidate A +20%, but the statistical nature of polling makes such an outcome very unlikely.
We introduce here an analogous concept for prediction markets, called the margin of inefficiency.
In words, the margin of inefficiency (MOI) is defined as the smallest deviation from the true price that is necessary to make a trade profitable.
More specifically, suppose a contract on Kamala Harris to win the 2024 U.S. presidential election is currently trading at $0.50, and suppose it was known with certainty that her true probability of winning is 53%. (For the sake of this discussion, we assume that this true probability of winning is publicly available information, known to everyone.)
Theoretically, each share purchased at $0.50 yields an expected profit of $0.03 due to the discrepancy between the price and the true underlying probability. In a perfectly efficient market, without any restrictions on trading or participation, the market price would adjust to $0.53. But in reality, markets can be slow to react, even when presented with unassailable information.
If, instead, the above market were available on PredictIt, then the decision to trade involves not only the $0.03 discrepancy between price and true probability, but also the commissions and fees associated with trading. Profitable trades on PredictIt are assessed a commission of 10% of realized profits, which for a contract bought at $0.50 (and which pays out at $1.00) equals a commission of $0.05. Therefore, the contract that is theoretically worth $0.03 in expected profit is, in practice, worth -$0.02. Based on this calculation, we say that 53% is within the margin of inefficiency of a contract currently trading at $0.50.
In fact, if we consider the additional 5% withdrawal fee assessed on all funds taken out of PredictIt, a deposit of $50 to purchase 100 shares for $0.50 each yields just $90.25 after withdrawal. Therefore, the theoretical $0.03 / share profit becomes a $0.0675 / share theoretical loss.
Based only on commissions and fees, the margin of inefficiency of a PredictIt contract priced at $0.50 is anywhere from +/- 5.0% to +/- 9.75% (depending on whether withdrawal fees are allocated to just one or a large number of profitable trades). This means that when Predictit shows a price of $0.50, the true underlying probability could be anywhere between 40.25% and 59.75%. In other words, the true probability would need to be less than 40.25% or larger than 59.75% in order for there to be a positive economic incentive to trade.
Note that this calculation does not include other factors that also contribute to inefficient market pricing, such as opportunity costs, time-value of money, and uncertainty about the true underlying probability. Opportunity costs include the value in keeping liquidity available to trade on more profitable opportunities should they arise. Such considerations will often cause traders to require a higher edge for bets that will take a longer time to settle. The time-value of money is especially relevant in political betting, for which betting markets are open for years before the event occurs. In high interest rate environments, this factor becomes especially pronounced.
By definition, the calculation of MOI assumes that the true probability is known with certainty. In reality, the true probability is not known, and traders often account for this uncertainty by requiring an additional margin of safety in order to trade. So, for example, even in the absence of any of the above frictions, a trader may choose not to trade at $0.50 if he believes the probability of the underlying event is 51% or 52%, because such a trade allows minimal room for error.
With all of these factors accounted for, the margin of inefficiency for PredictIt is easily north of 10% in either direction.
For comparison, Betfair charges a more modest 2% commission (on net market profits) and no withdrawal fee, meaning that a contract priced at $0.50 must deviate from the truth by at least $0.01 to warrant a profitable trade.
Polymarket, on the other hand, does not currently charge commissions, making its margin of inefficiency (based on fees alone) 0%.
With opportunity cost and time-value considerations taken into account, there is likely a built-in 0.50-1.00% inefficiency baked into any market price at any given time, even in very liquid markets.
The margin of inefficiency allows us to reason about betting markets whose prices don’t agree. For example, below are the prices across four betting markets as of October 7, 2024:
A first glance at these tell very different stories, with Donald Trump the favorite at Polymarket, Betfair, and Smarkets, and Harris the favorite on PredictIt. However, once the margin of inefficiency of these markets is factored in, we see that all markets are, technically, consistent with a narrow range of true probability values.
With one-month until the election, the time-value consideration is worth about 0.33% (assuming an annualized short-term risk-free interest rate of 4.8%). We assume opportunity cost contributes an additional 1.0%. We assess these equally to all markets and consider each market’s fee structure to arrive at the following MOI for each market:
From this, we can calculate a range of true probabilities for Harris consistent with each market, which reveals that the range of 46.3 - 47.2% falls within all of these intervals.
Therefore, applying the concept of margin of inefficiency, we could conclude that the consensus market forecast for Harris as of October 7 is between 46.27% - 47.23% and, conversely, the market consensus forecast for Trump is between 52.07% - 52.73%. (The market consensus forecast is defined as the range of probabilities that is consistent across all markets after accounting for margin of inefficiency.)
Further illustration of this concept, and validation of its consistency through time, is shown in the following graphic of market prices +/- their margin inefficiencies over several weeks in the campaign. Note that, despite short-term price fluctuations, all markets are consistent with one another, yielding a range of consensus market values, across all time points.
As the above demonstrates, there is logic in the markets. Price deviations among markets are often indicative of market inefficiencies, but not necessarily market irrationality. In particular, the inefficiencies that cause these price differences are more likely due to built-in structural factors, such as regulations and fees, rather than inconsistent use of information across platforms or persistent partisan bias among their respective user bases. By accounting for the structural component of price difference through the margin of inefficiency, we can make better sense of market prices across platforms to arrive at a coherent market consensus.